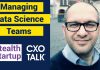
Sponsored by:
For senior leaders, managing data science is crucial to gain the most benefit from investments in AI and data analytics. Effective management involves people, culture, technology, and process. In this episode of CXOTalk, industry analyst, Michael Krigsman, interviews a top data scientist and business leader, who shares advice on how to create and manage a data science team.
Dr. Bülent Kiziltan is an AI executive and an accomplished scientist who uses artificial intelligence to create value in many business verticals and tackles diverse problems in disciplines ranging from the financial industry, healthcare, astrophysics, operations research, marketing, biology, engineering, hardware design, digital platforms, to art. He has worked at Harvard, NASA and MIT in close collaboration with pioneers of their respective fields. In the past 15+ years he has led data driven efforts in R&D and built multifaceted strategies for the industry. He has been a data science leader at Harvard and the Head of Deep Learning at Aetna leading and mentoring more than 200 scientists. In his current role, his data driven strategies with machine learning, analytics, engineering, marketing, and behavioral psychology components had a disruptive impact on a multi-billion dollar industry sector. Bülent’s previous appearance on CXOTalk was popular and engaging.
Topics discussed
- Unique aspects of data science
- From a management standpoint, what makes data science unique?
- Is there a distinction between managing a data science vs. an AI organization?
- Give us an overview of the unique challenges in managing data science?
- ROI and organizational expectations
- Who should own data science or AI in the organization?
- Where should data science report?
- Under Engineering, as a standalone organization, or someplace else?
- To what extent is data science an ROI or R&D basic research function?
- Is it imperative for corporate data science teams to maintain ongoing relationships with academics and external researchers?
- What are reasonable ROI expectations from the data science group?
- Talent profile
- What characteristics make a great data scientist?
- Do you need a technician, scientist, or business person?
- When hiring, what skills should you prioritize?
- Talent management
- Are there unique points on managing a team of data scientists?
- What about mentoring and ongoing training?
- What drives employee retention for data scientists?
- How can you make your workplace attractive to data scientists?
- How can a company entice data scientists when they are so much in demand?
TRANSCRIPT
Michael Krigsman: Today we’re talking about how to manage a team of data scientists. It’s a crucial topic. We’re speaking with Bülent Kiziltan, who is one of the most articulate and outspoken data scientists that I know.
Bülent Kiziltan: Thank you. Great to be on the show again, Michael.
Michael Krigsman: Give us a flavor of your background to set some context for us.
Bülent Kiziltan: I’m trained as a physicist and an astronomer. I have spent my career searching for neutron stars and black holes. During that pursuit, I used different aspects of apps like math and machine learning for more than 20 years. Now I’m applying those skills in the industry.
Michael Krigsman: All right, so I’m hoping that the topic we talk about today is going to be a little bit less complex than looking for neutrons and black holes but, hey, we’re talking about people, so maybe it’s more complex.
Bülent Kiziltan: That’s exactly what I was going to say.
Michael Krigsman: Okay. Bülent, what is unique about data science, unique about AI when it comes to building teams and managing teams?
Bülent Kiziltan: Right. First and foremost, it’s a new domain for the business and the industry. There is a lot of experimentation going on. The strategy, when it comes to managing, building data science teams and creating value with those teams is still in its infancy.
There is a lot of experimentation. Companies change strategies all the time. There is no single answer. There is no single right answer. Bigger companies versus smaller companies, industry domains matter a lot, and the culture is very, very important. I think we’re going to talk about that as well.
Michael Krigsman: Is there a distinction between managing data science and managing AI? I think there’s a lot of confusion between those two things.
Bülent Kiziltan: Right. Just a few days ago, I wanted to go online and look what people say on blogs about AI, data science, and analytics, what are the differences. Once you go through the blogs and the information that are in it, it’s really confusing. All of those posts have a certain level of truth and correctness in them but, mainly, it’s an area that’s very new to the industry.
AI is a more generic term that is considered more general an umbrella term that sits above data science, and data science typically would sit above analytics in terms of its comprehensiveness, if you will. But there is a lot of confusion. When it comes to etymology and how the context of the world changes, I think a culture and industry will play its part. So, it’s currently reshaping itself. There are overlapping things about all those three areas. There are things that are very different about all those three.
Michael Krigsman: From a management standpoint, do we need to think about them in very unique ways?
Bülent Kiziltan: I think, not based on whether it’s called AI, data science, or analytics, but I think what is more important in managing data science teams, if you will, is in what type of company and what type of domain you are operating in. Depending on the business objectives, I think one can come up with a more appropriate designation for the team. For instance, data science versus analytics.
Michael Krigsman: All right. I think that that sets the stage. Fundamentally, the issue is one of this being a new domain, and so we’re still trying to figure it out. Is that the correct sort of ground level beginning that we need to start from?
Bülent Kiziltan: That’s one important aspect. Another aspect of this is data science is science. It is still a hybrid of an academic culture and a business culture. Companies have a hard time hitting the right balance that aligns well with their business objectives. This is one area that we all are struggling and we’re experimenting with, hitting the right cultural balance within an operation.
I would say, one can come up with the most interesting strategy, but cultural eats strategy for breakfast. If your culture is not set right, you cannot execute on the strategy that you are thinking of.
Michael Krigsman: Let’s talk about the ROI and the organizational expectations for a team that’s being managed. Organizationally, where should a data science team or a data science department fit inside a company?
Bülent Kiziltan: If I were to think only like an academic, I would say they should be independent. Obviously, people who have been in the industry for a very long time, they have a different mindset. Depending on the company size and their business objectives, it could be considered as a supporting department and then many companies have data science operating on their engineering, but their business objectives are–I would imagine–more short-term.
AI has delivered on its promises. It’s creating a lot of ROI. Executives and the companies are well aware of what the ROI can be if analytics, operations, or data science operations can set their strategy independently of the engineering strategies because engineers have different priorities as opposed to analytics folks or data science folks. In my opinion, now we are at the stage where AI, data science, or analytics operations should be independently reporting to the board or be represented at the C-level at least regardless of the size of the company.
Michael Krigsman: I want to remind everybody that we’re speaking with Bülent Kiziltan, and we’re talking about how to manage AI and data science organizations; what are the unique aspects of this?
Bülent, the kind of expectations that companies have of their AI departments or their data science departments, you made a distinction between the business expectations and the technology or the engineering expectations. Can you elaborate on that?
Bülent Kiziltan: Everybody knows there is a certain level of hype that comes with AI or data science. Early, when this hype started, we had seen some justified skepticism from high-level business executives because the teams that were formed were not, essentially, executing or delivering the ROI. I would say there were some unrealistic expectations at the beginning but, right now, we are at the level where we can utilize more mature tools. The talent pool has diversified itself. Still, there is not enough talent out there, but we hire data scientists from very different profiles.
Data science, I would say that it is more of a creative process than an engineering process. Engineering processes require creativity as well, but I think that that creative aspect is very dominant in data science processes. An engineering mindset typically has an output and input and they are, generically speaking, trying to optimize that whole process whereas, in data science, if managed properly and has an aligned vision with the company, is about discovery, extracting new information. That is very different from a sole engineering operations perspective.
This is why I think companies who are trying to make an impact, who are trying to come up with disruptive innovation on the large scale or on the small scale, I think, are moving in the direction where data science operations now are led by domain experts that really have done data science, have written code, and they’re leading the data science operations by example. This is why we have all sorts of titles floating around. That leader type is also very scarce right now, but people and leaders who are domain experts in data science or AI but also have a business acumen and experience are the leaders that are highly sought after today.
Michael Krigsman: You mentioned that data science needs to be aligned with the goals of the organization.
Bülent Kiziltan: Right.
Michael Krigsman: Please, talk about that. That seems like a very crucial dimension here.
Bülent Kiziltan: There are, I think, two extremes when it comes to building a data science strategy. One extreme is the academic mindset where you do research for long-term impact. The other extreme is the short-term pragmatism that comes with short-term deliverables in the business setting.
I think, in data science operations, the balance has to be set right to align with the business objectives of any company. There are larger companies such as Google and Facebook which have the resources to make mid-term and long-term investments only. They have research teams that really operate like academic institutions. Most of the companies don’t have those resources. They don’t have those objectives.
Any leader who is coming in, I think, has to first and foremost identify the business objectives and what sort of short-term, midterm, and long-term deliverables he can deliver to the board in order to justify the operation’s existence. This is very important.
On the other side, and we see that more often than not, AI or analytics operations are managed by non-domain experts. I would call them managers. There are some pragmatic reasons why you would want a person that doesn’t have a data science background but has really the business experience, especially in larger companies, mainly because the value that one can create is most often hindered by the internal dynamics and the stakeholders. A leader that goes into a bigger company has to really consider the balance of different stakeholders and convince them. That comes with business experience.
I think the right balance is somewhere in between where you really deliver on the short-term. There are lots of low hanging fruits in all sorts of business settings, especially in larger companies. A smart leader would focus on delivering the short-term and invest in the long-term.
Michael Krigsman: Given the importance of aligning the data science efforts–and you could say the same thing for AI–can you share with us any examples where the business goals, where the data science efforts were not aligned with the business goals? What’s the kind of outcome that then happens?
Bülent Kiziltan: I will talk about what we generalists see in larger companies today is data science and analytics efforts are led by non-domain experts. They don’t know much about data science itself, but they know really how to manage groups, how to maybe build groups, and how to talk to different stakeholders internally.
Through that relationship, they can justify their existence and large budgets by delivering on the short-term.
When you have an AI manager, if you will, I wouldn’t call them leaders. If you have a manager that has a sole business objective and business background, they will have the shortsightedness that comes with the business pragmatism. They will go after the low hanging fruit only because this is how they have been operating and delivering it to the business executives.
Data science operations, especially when it comes to sustaining the value that AI promises, requires long-term investment. One of that investment is to attract talent and to retain that talent. If you have a data science operation that focuses on short-term goals only without giving the data scientists creative space, those folks will be very difficult to be retained in any type of operations. In that relationship, an employer has to ask what type of value do I bring to the table and do I invest in the continual mentorship and training of the data scientists.
In different domains, the domain knowledge that the company has adds to the employee. But, within data science, one of the nice things about data science is it’s somewhat domain agnostic. So, when a data scientist comes onboard, they will build the required domain knowledge, whether you’re in healthcare or in finance, about what they do, and the value that they create is not directly related to that domain expertise. So, they know their worth and they can switch from one domain to another.
As an employer, the companies have to really consider how to retain that talent. There are methods one can do that. There is a clear ROI case to build a culture in which data scientists collaborate rather than compete, it’s more human-centric, and there is an academic aspect to that operation. It’s because if you are looking just at the skillset of data scientists and work them 150%, that skillset will become obsolete in six months. Continual training and an environment in which intellectually they get enriched is essential in any type of data science operations, in my opinion.
Michael Krigsman: Let’s shift gears here and talk about data science talent. First off, why is this such an important issue?
Bülent Kiziltan: It’s a new domain and there are a lot of people who are trying to come into that domain from very diverse perspectives, very diverse trainings. I think all of them bring an important aspect from their own domain into the creative process of doing data science. Having folks with a psychology background, having a data scientist coming from a math background or an astrophysics background, they all bring in interesting ideas.
As I said, data science is more about the creative process and problem solving than just using certain tools. To know how to use certain tools is important, but what is more important is problem-solving skills. Each diverse background brings an interesting aspect and perspective to the problem-solving process. So, that is very important.
One of the negative sides of being in a new domain is every data scientist is above average in one of those three aspects when it comes to statistics, code writing, or the domain expertise or when it comes to contributing to a problem solving a certain domain. There has to be a continual training for data scientists, and I value very much diverse backgrounds. That is one of my approaches to building data science teams. Diversity is very important.
Michael Krigsman: You’ve mentioned culture several times now as being really important. We have a question from Twitter. Arsalan Khan asks, “How do you create an AI-focused culture when employees are fearful that their jobs will be taken away by that AI?”
Bülent Kiziltan: By that AI? Well, the fear that AI will come and take jobs is largely unjustified, I think. Some folks who are popularizing AI, they come up with figures and claim that AI will take away jobs. I ask them, “What’s the data? How do you justify that claim?”
I don’t know whether AI will take away jobs, but what I know for sure is the demographics in how the labor market is going to look in five years will be very different from today. Just looking back in innovations that are similar to AI, like the invention of electricity or engines in the past, it changed the labor market dramatically but didn’t diminish the labor market in numbers. I don’t think so.
I don’t think that AI, in general, will have a negative impact on the job security for the future. I think that fear is not justified. this has to be addressed.
Setting the culture, I think that fear is not the most important issue. The most important issue is to create an environment which is collaborative and human-centric. It’s intellectually very rich mainly because the problems that we’re facing today are very complex and we need creative ideas to solve those problems. Data science, AI, and machine learning is providing very powerful tools to solve those problems.
People coming with all sorts of different backgrounds are bringing in something that’s valuable. As a leader, we have to make sure that each individual’s opinion is valued. The merit of the opinion wins over the title. This is my approach to things.
Ideas are very important and data science is a new domain. None of us are formally trained in data science. Everybody brings in something different which has value.
Michael Krigsman: What about the relationship between academics and data science inside, say, especially large companies, but I think it’s probably equally true for smaller venture-funded startups.
Bülent Kiziltan: Yes. The bleeding edge know-how is still created and produced within academic settings or in larger companies that operate like academic institutions. We have to consider this if you want to continue and sustain the value that data science creates for companies. I’m an advocate to continue the relationship with my colleagues from the academic institutions and building really productive relationships both ways.
There is a lot that we can learn from folks that are operating in academia. I still read The Archive every day and see what’s coming out in my own domain, in both domains, in astrophysics and in data science or AI-related fields. But also, create a relationship with them that gives them incentives to contribute to solving problems in the industry.
Some bigger companies find different solutions for that where they fund academics full time or they give them the resources so that they don’t have to think about bringing in grants and focus on problem-solving, which is their main job. But in any setting, in smaller companies or bigger companies, I think there has to be some sort of an ongoing productive relationship with the academic world in order to sustain the value that AI is bringing today.
Michael Krigsman: What about smaller companies? How do smaller companies manage? It’s clear with a large company. They have the resources to do that, but what about a smaller company?
Bülent Kiziltan: Smaller companies actually hire folks directly with very strong academic backgrounds. Larger companies, on the other hand, because they have internal dynamics and silos, they typically hire people who are not domain experts in data science but are business managers. I don’t think startups have a problem in building that relationship very informally and casually with academic institutions because this is their main source where they hire that talent. Whereas, at bigger companies, they have their own problems bringing academics into their operations.
Michael Krigsman: It sounds like you’re a big fan of the kind of work that’s done, innovation–put it that way–inside smaller companies.
Bülent Kiziltan: That’s right. I mean I’ve operated and worked in both domains, if you will in the startup space and in bigger companies. Both have pros and cons, positive and negative aspects to their operations. But, when it comes to innovation, I think moving quickly is very important and, startups, they don’t have the stigma of having different silos. They are very fluid in terms of hierarchy, so they can provide and make that impact really quickly.
Time is an important asset today, especially when it comes to AI. Things are changing on a weekly basis, so we have to be quick. Having larger companies having a lot of people to manage, internal dynamics to overcome is a lot of wasted time and resources for bigger companies.
This is why I’ve seen a trend where bigger companies are acquiring smaller companies for their AI operations or they are having a more organic merger with different companies. Smaller companies definitely have their advantages when it comes to innovation.
Michael Krigsman: The challenge that small companies face is they have speed, they can move quickly, but they often don’t have the resources that large companies do. And so, what are you seeing inside smaller companies when it comes to AI and data science to let them overcome the lack of resources?
Bülent Kiziltan: That’s correct. The lack of resources is one of the disadvantages smaller companies have. One important resource that bigger companies have is access to data. Data is currency when it comes to doing data science.
Bigger companies cannot move fast, whereas smaller companies can move really quickly. Depending on the problem that they’re working on, sometimes working with smaller teams is more advantageous than working with bigger teams, to be honest. As long as smaller companies have access to data, I think the day-to-day operations, the hardware requirements are not that enormous and you don’t need an army of data scientists to address a certain problem or come up with an interesting solution. I think the asset that bigger companies have is the data that they’re owning and smaller companies have the speed, so I encourage companies to build synergistic relationships in that regard.
Michael Krigsman: That’s a really interesting point; smaller companies have speed and larger companies have data. That’s a uniquely AI focused proposition today.
Bülent Kiziltan: I think, yes, it’s very different from what we’ve seen until the emergence of data science, how that relationship worked. I think startups today have a lot of leverage doing data science and AI as long as they have access to that data. Sometimes the data is being produced in academic settings, and this is why we see a lot of academics and professors who are building companies, startups, and moving quickly and producing value that way.
Michael Krigsman: Let’s talk about finding data scientists. When I speak with executives, the lack of resources seems to be a constant complaint.
Bülent Kiziltan: There’s definitely a lack of talent. That’s for sure. I think an important part of the searching process is how the recruitment process works.
What I’ve seen in companies, especially bigger companies, their recruitment teams still use the old fashioned way of going after data scientists and, most of the time, they’re missing the real talent. They’re searching for certain keywords. They’re looking for a certain type of experience that is not out there and is not essentially required in the data science operations.
I think reforming, restructuring, and retraining the HR and the recruiters is an important part in proactively going after the data science talent. They are looking in the wrong places most of the time.
Michael Krigsman: What are the wrong places and what are the right places?
Bülent Kiziltan: How they scan CVs, for instance, they’re looking for certain keywords like Python and the type of learning anybody can put on their CV. But it’s very difficult to gauge the creative aspect of a certain individual, so there are metrics that one can use to gauge the creativity that an individual has. But I’m a face-to-face person, so a five-minute face-to-face meeting is worth any type of strategy in recruitment. Meeting a person face-to-face and talking to them gives a lot of insight into what type of person they are, how they go after a certain type of problem. Many companies are filtering based on certain skill sets, and I think that’s the wrong way to go about searching talented data scientists.
Michael Krigsman: The problem is many recruiters are searching for specific languages, as an example, none of which can point to creativity, and creativity is so essential.
Bülent Kiziltan: That’s right. In data science, in my opinion, what language you use, in most of the use cases, the language is not relevant. I advise them to use the language that they are most comfortable with.
When it comes to certain tools, they are looking for tools. Tools are being produced on a weekly basis. They are dynamically changing. What you put on your CV today is becoming irrelevant in a couple of months and there is a new tool. So, what I look for is creativity and the willingness to learn rather than a certain keyword on your CV. I think recruiters, most of them are going about this the wrong way.
Michael Krigsman: Is that any different from hiring software developers?
Bülent Kiziltan: Yes, it is somewhat different. In software development, things are more mature, experience is very important, and the process is really well defined, whereas data science operations, as I said, is still in its experimentation and there is no single answer. You can take two very similar companies, based on their leadership and management style, you have to hire different people that align better with the culture of the particular company. So, it’s a very dynamic domain and one cannot go about this in a deterministic manner. you really have to meet the candidate and talk to them.
Obviously, screening is very important, and we really appreciate the efforts of recruiters and how they help us in the hiring process. But we have to go about data science hiring in a different way.
Michael Krigsman: What makes a company attractive to a data scientist, if you want to set up the right kind of environment to attract folks?
Bülent Kiziltan: Yeah. Everybody has a different background. They have different experiences that they bring to the table. For me, for instance, right now, I think what I focus on is the culture. The culture has to be right for the data scientists. When I mean right, I mean a place where they can continually learn, where they can bring interesting ideas to the table, and the ideas are being valued.
The merit of the idea is more important than the title of a person and an intellectually rich environment. Then, obviously, the problems that they’re working on, how interesting they are is important for people to choose one company over another.
If a company doesn’t set the culture right, as I said, the turnover will be very high, which is a very high cost for companies. That high turnover will make a data scientist question whether it’s a right choice or not. If a company has a high number of turnover, they have to really rethink their strategy and approach to data science, in general, in my opinion.
Michael Krigsman: Arsalan Khan, on Twitter, makes a really interesting point. He says, “Very often HR is not equipped to hire data scientists because they don’t know enough to evaluate who is good and who is not.” I think this gets back to the point that you raised earlier that searching, scanning for keywords on a CV or a resume does not help you evaluate the creativity or the potential of that person at all.
Bülent Kiziltan: Right and wrong. Certainly, he is right when it comes to domain expertise. They don’t. But recruiters and HR is not required to be a domain expert when they are screening the candidate. How they screened in the past based on keywords regarding domains that are mature like software development or certain types of engineering should be very different than looking for a particular type and profile of a data scientist is what I’m saying. They don’t require domain expertise to do that.
Michael Krigsman: What you’re essentially saying is you need to be looking for innovation potential.
Bülent Kiziltan: Right. For instance, has a person operated in different domains and still remain productive? I think that’s an important metric to gauge the learning willingness of a candidate and the creativity of a candidate.
If a person comes from an academic background, have they produced academic work in different topics, for instance, is an important metric. If they have been in the industry, have they been in different domains? If they have remained in the same domain for 15 years, that makes them a really deep domain expert, but will this make a person the right candidate for data science? I don’t think so.
Michael Krigsman: Gus Bekdash, on Twitter, makes a really interesting point. He says, “One principle that’s helped him in the past is looking at the kinds of problems that a person has solved. What do you think about that?
Bülent Kiziltan: Yes, but not everybody has the luxury to choose the problems that they want to work on, both in the academic setting and in the industry setting as well. I kind of evaluate this more dynamically. I look into the problem. It has a certain weight. Certainly, they have some leverage in choosing it. But once they’re in an operation, typically they are assigned certain problems, and I look at what they have brought to the solution.
Michael Krigsman: That’s the key. It’s not just the problem they’re working on but the nature of the solution that they’re applying.
Bülent Kiziltan: Absolutely. This also will give insight into how you can build your CV and make it more transparent to the hiring manager. You should probably include some of the relevant solutions that you brought to a certain type of problem. I think that is very relevant.
Michael Krigsman: Yeah, that’s a very, very interesting point. What you’re really trying to do is help the hiring manager gain insight into the way that you think.
Bülent Kiziltan: Yes, that’s a very tough one. It’s a challenge for everyone, including recruiters, including hiring managers. It is an area that we also keep experimenting. Some companies, they have online challenges, coding challenges, which work or it doesn’t work. I don’t like online challenges, but I like to sit down with candidates and stay in front of a board and have a conversation, focus on some problem, and see how they think.
This is a very subjective process. I agree with that. This is why data science candidates should not be discouraged if you’re turned down by certain companies because everybody has a different approach. It doesn’t reflect their worth, so it’s a very subjective process.
Michael Krigsman: One of the issues, I think, that comes up, especially in larger companies, and you alluded to this earlier, is that the aspect of data science can get lost relative to the importance of process, corporate flow, desire to look good, and so forth.
Bülent Kiziltan: That’s right. This is why choosing the right leader is very important. My approach to leadership is to lead by example. I would imagine a leader who really has written code and models and knows about a process itself is an important asset in this regard to hit the right balance when it comes to focusing on short-term business objectives and deliverables and then also investing in the long-term future.
If the manager of a certain type of data science operations is focused only on one aspect, whether it be R&D for the long-term or just short-term deliverables, I think will face some hardship in the midterm. So, in big companies, as I said, because the domain experts have operated mainly in academic settings, have somewhat limited business experience compared to managers, consultants, or executives who have been in the industry for decades. You cannot compare their business experience, for sure. I think what is more important is to bring a person that has a reputable domain expertise and has business acumen leadership skills as well and invest into that person if you want to break ground using AI.
Michael Krigsman: The ultimate issue is, as you say, how are we breaking ground? Right? That’s very different from, say, developing traditional enterprise software business applications.
Bülent Kiziltan: Yes, breaking ground. Everybody has a different approach to breaking ground or doing disruptive innovation. But what I’ve seen in many companies is, when it comes to AI, when you look at the nuts and bolts of what they actually do, sometimes what they promise is actually not there. This has to do with not having the right talent or not having the right level of investment into AI, but they want to move in the right direction, so that’s an understandable position to take where they advertise a vision and they are trying to build a team that can align with that vision, which is very important.
It’s, I think, critically important to have the right leader that is setting the tone for the culture. A leader versus a manager is very important. Go after the leaders that have some domain expertise.
Michael Krigsman: What about at the senior level inside a company, at the board level, the senior executive level, folks who are setting the goals for the business? How does that translate down into AI efforts and data science efforts?
Bülent Kiziltan: Again, I think the leader who is managing data science operations is critically important here. The person has to be an educator, coming from a background in which they have operated at a level where they can break down technical stuff to simple terms. This is how a leader that is operating within data science has to talk with the board. You cannot just go about a deep learning network and talk about backpropagation in the board meeting.
With startup companies, this may not be a big problem. But with bigger companies, I think the communication skill plays a critical role when the data science leader comes to the meeting and talks about the objectives that they have and how it aligns with the business objectives. On the other side of the table, it requires an executive board and a CEO that is willing to learn.
We had this conversation before. We live at a time in which every business leader has to be articulate and learn some aspects of machine learning and AI in order to make the right decision. If it’s a person that is not open to learning, that doesn’t value the best ideas but is looking for a certain type of presentation or looks at the fonts and the colors of a PowerPoint rather than look at the content of the PowerPoint, I think that’s the place where that relationship can break ground or fail.
Michael Krigsman: Isn’t this really just like It folks? How is this different from the historical problem that technical folks have had communicating with businesspeople? It seems like that’s the same issue.
Bülent Kiziltan: When it comes to communicating, I think, yeah, the problems are similar, but the business objectives, the investment types, and the strategies are very different. There will be a certain structure of communication and a certain expectation from the board that they are used to.
The leader, the data science leader either conforms into this expectation, I think which is the wrong way to do. The data science leader has to be bold but also has to align the business objectives of the data science operations with the board. What the data science leader brings to the table will be very different from an IT leader.
Michael Krigsman: As we finish up, can you describe those differences between managing an IT function like the CIO, for example, and managing an AI or data science operation?
Bülent Kiziltan: I don’t want to talk too much about the IT folks because it’s not my area of expertise, I would say, per se. But I would imagine that the IT infrastructure is much more mature. The day-to-day expectation, how they set up certain goals is more a set as opposed to a domain, such as data science, in which the leader has to reinvent himself or herself on a weekly basis.
You basically have to encourage your team to fail but fail quickly rather than in IT. That margin for failure might be very, very small as opposed to in data science. We are experimenting quite a bit, and so there is a certain overhead that comes with that experimentation.
We face different types of challenges, but by no means do I want to say that IT leadership is less challenging. We all face different challenges.
Michael Krigsman: That is certainly the truth. As we finish up, Bülent, any final thoughts on this topic?
Bülent Kiziltan: Data science, AI, machine learning, and analytics is a great place to be. I see a lot of younger people who are moving into the field. I want to encourage them to move in. Most of the people are coming in, they are suffering from a syndrome where they think they are not properly trained as a data scientist, so they are less worth as opposed to more formally trained computer scientists. I don’t think this is the case. A diverse background has a lot of business value.
From a leaders perspective, creating that creative space for data scientists, regardless of their level, produces a lot of ROI. It has business value that comes with it. I encourage the leaders to create that space for the incoming data scientists. Invest into their continual training if you want to sustain the value that you have produced in the short-term.
Michael Krigsman: Okay. Bülent Kiziltan, thank you so much for joining us today. It’s been a very fascinating discussion.
Bülent Kiziltan: It’s been a pleasure, Michael.
Michael Krigsman: You have been watching this interesting discussion on how we manage data science and artificial intelligence operations. Thanks for watching. Please subscribe on YouTube to our YouTube challenge and go to CXOTalk.com to see more videos. Be sure to subscribe to our newsletter. Thanks, everybody. Have a great day. Check out our videos and we’ll see you next time. Bye-bye.
Ai
via https://www.AiUpNow.com
Josh Johnson, Khareem Sudlow