Fbeta-measure is a configurable single-score metric for evaluating a binary classification model based on the predictions made for the positive class.
The Fbeta-measure is calculated using precision and recall.
Precision is a metric that calculates the percentage of correct predictions for the positive class. Recall calculates the percentage of correct predictions for the positive class out of all positive predictions that could be made. Maximizing precision will minimize the false-positive errors, whereas maximizing recall will minimize the false-negative errors.
The F-measure is calculated as the harmonic mean of precision and recall, giving each the same weighting. It allows a model to be evaluated taking both the precision and recall into account using a single score, which is helpful when describing the performance of the model and in comparing models.
The Fbeta-measure is a generalization of the F-measure that adds a configuration parameter called beta. A default beta value is 1.0, which is the same as the F-measure. A smaller beta value, such as 0.5, gives more weight to precision and less to recall, whereas a larger beta value, such as 2.0, gives less weight to precision and more weight to recall in the calculation of the score.
It is a useful metric to use when both precision and recall are important but slightly more attention is needed on one or the other, such as when false negatives are more important than false positives, or the reverse.
In this tutorial, you will discover the Fbeta-measure for evaluating classification algorithms for machine learning.
After completing this tutorial, you will know:
- Precision and recall provide two ways to summarize the errors made for the positive class in a binary classification problem.
- F-measure provides a single score that summarizes the precision and recall.
- Fbeta-measure provides a configurable version of the F-measure to give more or less attention to the precision and recall measure when calculating a single score.
Discover SMOTE, one-class classification, cost-sensitive learning, threshold moving, and much more in my new book, with 30 step-by-step tutorials and full Python source code.
Let’s get started.
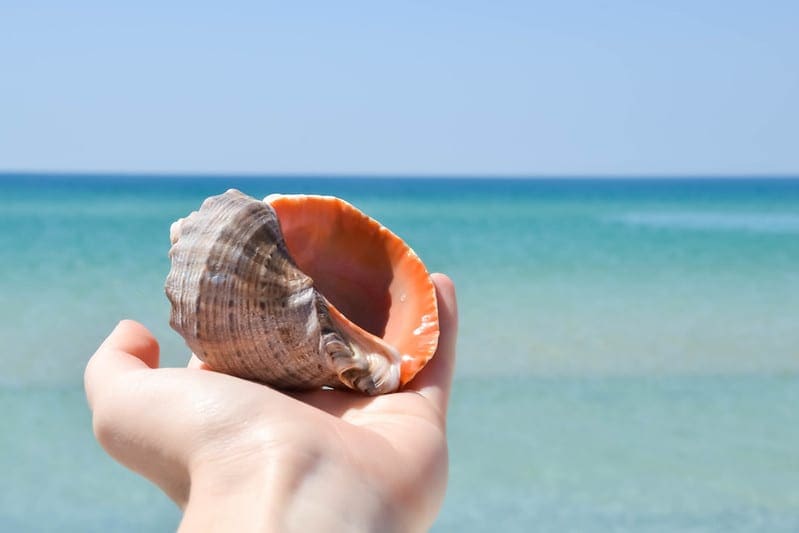
A Gentle Introduction to the Fbeta-Measure for Machine Learning
Photo by Marco Verch, some rights reserved.
Tutorial Overview
This tutorial is divided into three parts; they are:
- Precision and Recall
- Confusion Matrix
- Precision
- Recall
- F-Measure
- Worst Case
- Best Case
- 50% Precision, Perfect Recall
- Fbeta-Measure
- F1-Measure
- F0.5 Measure
- F2 Measure
Precision and Recall
Before we can dive into the Fbeta-measure, we must review the basics of the precision and recall metrics used to evaluate the predictions made by a classification model.
Confusion Matrix
A confusion matrix summarizes the number of predictions made by a model for each class, and the classes to which those predictions actually belong. It helps to understand the types of prediction errors made by a model.
The simplest confusion matrix is for a two-class classification problem, with negative (class 0) and positive (class 1) classes.
In this type of confusion matrix, each cell in the table has a specific and well-understood name, summarized as follows:
| Positive Prediction | Negative Prediction Positive Class | True Positive (TP) | False Negative (FN) Negative Class | False Positive (FP) | True Negative (TN)
The precision and recall metrics are defined in terms of the cells in the confusion matrix, specifically terms like true positives and false negatives.
Precision
Precision is a metric that quantifies the number of correct positive predictions made.
It is calculated as the ratio of correctly predicted positive examples divided by the total number of positive examples that were predicted.
- Precision = TruePositives / (TruePositives + FalsePositives)
The result is a value between 0.0 for no precision and 1.0 for full or perfect precision.
The intuition for precision is that it is not concerned with false negatives and it minimizes false positives. We can demonstrate this with a small example below.
# intuition for precision from sklearn.metrics import precision_score # no precision y_true = [0, 0, 0, 0, 0, 1, 1, 1, 1, 1] y_pred = [0, 0, 0, 0, 0, 0, 0, 0, 0, 0] score = precision_score(y_true, y_pred) print('No Precision: %.3f' % score) # some false positives y_true = [0, 0, 0, 0, 0, 1, 1, 1, 1, 1] y_pred = [0, 0, 0, 1, 1, 1, 1, 1, 1, 1] score = precision_score(y_true, y_pred) print('Some False Positives: %.3f' % score) # some false negatives y_true = [0, 0, 0, 0, 0, 1, 1, 1, 1, 1] y_pred = [0, 0, 0, 0, 0, 0, 0, 1, 1, 1] score = precision_score(y_true, y_pred) print('Some False Negatives: %.3f' % score) # perfect precision y_true = [0, 0, 0, 0, 0, 1, 1, 1, 1, 1] y_pred = [0, 0, 0, 0, 0, 1, 1, 1, 1, 1] score = precision_score(y_true, y_pred) print('Perfect Precision: %.3f' % score)
Running the example demonstrates calculating the precision for all incorrect and all correct predicted class labels, which shows no precision and perfect precision respectively.
An example of predicting some false positives shows a drop in precision, highlighting that the measure is concerned with minimizing false positives.
An example of predicting some false negatives shows perfect precision, highlighting that the measure is not concerned with false negatives.
No Precision: 0.000 Some False Positives: 0.714 Some False Negatives: 1.000 Perfect Precision: 1.000
Recall
Recall is a metric that quantifies the number of correct positive predictions made out of all positive predictions that could have been made.
It is calculated as the ratio of correctly predicted positive examples divided by the total number of positive examples that could be predicted.
- Recall = TruePositives / (TruePositives + FalseNegatives)
The result is a value between 0.0 for no recall and 1.0 for full or perfect recall.
The intuition for recall is that it is not concerned with false positives and it minimizes false negatives. We can demonstrate this with a small example below.
# intuition for recall from sklearn.metrics import recall_score # no recall y_true = [0, 0, 0, 0, 0, 1, 1, 1, 1, 1] y_pred = [0, 0, 0, 0, 0, 0, 0, 0, 0, 0] score = recall_score(y_true, y_pred) print('No Recall: %.3f' % score) # some false positives y_true = [0, 0, 0, 0, 0, 1, 1, 1, 1, 1] y_pred = [0, 0, 0, 1, 1, 1, 1, 1, 1, 1] score = recall_score(y_true, y_pred) print('Some False Positives: %.3f' % score) # some false negatives y_true = [0, 0, 0, 0, 0, 1, 1, 1, 1, 1] y_pred = [0, 0, 0, 0, 0, 0, 0, 1, 1, 1] score = recall_score(y_true, y_pred) print('Some False Negatives: %.3f' % score) # perfect recall y_true = [0, 0, 0, 0, 0, 1, 1, 1, 1, 1] y_pred = [0, 0, 0, 0, 0, 1, 1, 1, 1, 1] score = recall_score(y_true, y_pred) print('Perfect Recall: %.3f' % score)
Running the example demonstrates calculating the recall for all incorrect and all correct predicted class labels, which shows no recall and perfect recall respectively.
An example of predicting some false positives shows perfect recall, highlighting that the measure is not concerned with false positives.
An example of predicting some false negatives shows a drop in recall, highlighting that the measure is concerned with minimizing false negatives.
No Recall: 0.000 Some False Positives: 1.000 Some False Negatives: 0.600 Perfect Recall: 1.000
Now that we are familiar with precision and recall, let’s review the F-measure.
Want to Get Started With Imbalance Classification?
Take my free 7-day email crash course now (with sample code).
Click to sign-up and also get a free PDF Ebook version of the course.
F-Measure
Precision and recall measure the two types of errors that could be made for the positive class.
Maximizing precision minimizes false positives and maximizing recall minimizes false negatives.
F-Measure or F-Score provides a way to combine both precision and recall into a single measure that captures both properties.
- F-Measure = (2 * Precision * Recall) / (Precision + Recall)
This is the harmonic mean of the two fractions.
The result is a value between 0.0 for the worst F-measure and 1.0 for a perfect F-measure.
The intuition for F-measure is that both measures are balanced in importance and that only a good precision and good recall together result in a good F-measure.
Worst Case
First, if all examples are perfectly predicted incorrectly, we will have zero precision and zero recall, resulting in a zero F-measure; for example:
# worst case f-measure from sklearn.metrics import f1_score from sklearn.metrics import precision_score from sklearn.metrics import recall_score # no precision or recall y_true = [0, 0, 0, 0, 0, 1, 1, 1, 1, 1] y_pred = [1, 1, 1, 1, 1, 0, 0, 0, 0, 0] p = precision_score(y_true, y_pred) r = recall_score(y_true, y_pred) f = f1_score(y_true, y_pred) print('No Precision or Recall: p=%.3f, r=%.3f, f=%.3f' % (p, r, f))
Running the example, we can see that no precision or recall results in a worst-case F-measure.
No Precision or Recall: p=0.000, r=0.000, f=0.000
Given that precision and recall are only concerned with the positive class, we can achieve the same worst-case precision, recall, and F-measure by predicting the negative class for all examples:
# another worst case f-measure from sklearn.metrics import f1_score from sklearn.metrics import precision_score from sklearn.metrics import recall_score # no precision and recall y_true = [0, 0, 0, 0, 0, 1, 1, 1, 1, 1] y_pred = [0, 0, 0, 0, 0, 0, 0, 0, 0, 0] p = precision_score(y_true, y_pred) r = recall_score(y_true, y_pred) f = f1_score(y_true, y_pred) print('No Precision or Recall: p=%.3f, r=%.3f, f=%.3f' % (p, r, f))
Given that no positive cases were predicted, we must output a zero precision and recall and, in turn, F-measure.
No Precision or Recall: p=0.000, r=0.000, f=0.000
Best Case
Conversely, perfect predictions will result in a perfect precision and recall and, in turn, a perfect F-measure, for example:
# best case f-measure from sklearn.metrics import f1_score from sklearn.metrics import precision_score from sklearn.metrics import recall_score # perfect precision and recall y_true = [0, 0, 0, 0, 0, 1, 1, 1, 1, 1] y_pred = [0, 0, 0, 0, 0, 1, 1, 1, 1, 1] p = precision_score(y_true, y_pred) r = recall_score(y_true, y_pred) f = f1_score(y_true, y_pred) print('Perfect Precision and Recall: p=%.3f, r=%.3f, f=%.3f' % (p, r, f))
Running the example, we can see that perfect precision and recall results in a perfect F-measure.
Perfect Precision and Recall: p=1.000, r=1.000, f=1.000
50% Precision, Perfect Recall
It is not possible to have perfect precision and no recall, or no precision and perfect recall. Both precision and recall require true positives to be predicted.
Consider the case where we predict the positive class for all cases.
This would give us 50 percent precision as half of the predictions are false positives. It would give us perfect recall because we would no false negatives.
For the balanced dataset we are using in our examples, half of the predictions would be true positives, half would be false positives; therefore, the precision ratio would be 0.5 or 50 percent. Combining 50 percept precision with perfect recall will result in a penalized F-measure, specifically the harmonic mean between 50 percent and 100 percent.
The example below demonstrates this.
# perfect precision f-measure from sklearn.metrics import f1_score from sklearn.metrics import precision_score from sklearn.metrics import recall_score # perfect precision, 50% recall y_true = [0, 0, 0, 0, 0, 1, 1, 1, 1, 1] y_pred = [1, 1, 1, 1, 1, 1, 1, 1, 1, 1] p = precision_score(y_true, y_pred) r = recall_score(y_true, y_pred) f = f1_score(y_true, y_pred) print('Result: p=%.3f, r=%.3f, f=%.3f' % (p, r, f))
Running the example confirms that we indeed have 50 percept precision and perfect recall, and that the F-score results in a value of about 0.667.
Result: p=0.500, r=1.000, f=0.667
Fbeta-Measure
The F-measure balances the precision and recall.
On some problems, we might be interested in an F-measure with more attention put on precision, such as when false positives are more important to minimize, but false negatives are still important.
On other problems, we might be interested in an F-measure with more attention put on recall, such as when false negatives are more important to minimize, but false positives are still important.
The solution is the Fbeta-measure.
The Fbeta-measure measure is an abstraction of the F-measure where the balance of precision and recall in the calculation of the harmonic mean is controlled by a coefficient called beta.
- Fbeta = ((1 + beta^2) * Precision * Recall) / (beta^2 * Precision + Recall)
The choice of the beta parameter will be used in the name of the Fbeta-measure.
For example, a beta value of 2 is referred to as F2-measure or F2-score. A beta value of 1 is referred to as the F1-measure or the F1-score.
Three common values for the beta parameter are as follows:
- F0.5-Measure (beta=0.5): More weight on precision, less weight on recall.
- F1-Measure (beta=1.0): Balance the weight on precision and recall.
- F2-Measure (beta=2.0): Less weight on precision, more weight on recall
The impact on the calculation for different beta values is not intuitive, at first.
Let’s take a closer look at each of these cases.
F1-Measure
The F-measure discussed in the previous section is an example of the Fbeta-measure with a beta value of 1.
Specifically, F-measure and F1-measure calculate the same thing; for example:
- F-Measure = ((1 + 1^2) * Precision * Recall) / (1^2 * Precision + Recall)
- F-Measure = (2 * Precision * Recall) / (Precision + Recall)
Consider the case where we have 50 percept precision and perfect recall. We can manually calculate the F1-measure for this case as follows:
- F-Measure = (2 * Precision * Recall) / (Precision + Recall)
- F-Measure = (2 * 0.5 * 1.0) / (0.5 + 1.0)
- F-Measure = 1.0 / 1.5
- F-Measure = 0.666
We can confirm this calculation using the fbeta_score() function in scikit-learn with the “beta” argument set to 1.0.
The complete example is listed below.
# calculate the f1-measure from sklearn.metrics import fbeta_score from sklearn.metrics import precision_score from sklearn.metrics import recall_score # perfect precision, 50% recall y_true = [0, 0, 0, 0, 0, 1, 1, 1, 1, 1] y_pred = [1, 1, 1, 1, 1, 1, 1, 1, 1, 1] p = precision_score(y_true, y_pred) r = recall_score(y_true, y_pred) f = fbeta_score(y_true, y_pred, beta=1.0) print('Result: p=%.3f, r=%.3f, f=%.3f' % (p, r, f))
Running the example confirms the perfect precision and 50 percent recall and an F1-measure of 0.667, confirming our calculation (with rounding).
This F1-measure value of 0.667 matches the F-measure calculated for the same scenario in the previous section.
Result: p=0.500, r=1.000, f=0.667
F0.5-Measure
The F0.5-measure is an example of the Fbeta-measure with a beta value of 0.5.
It has the effect of raising the importance of precision and lowering the importance of recall.
If maximizing precision minimizes false positives, and maximizing recall minimizes false negatives, then the F0.5-measure puts more attention on minimizing false positives than minimizing false negatives.
The F0.5-Measure is calculated as follows:
- F0.5-Measure = ((1 + 0.5^2) * Precision * Recall) / (0.5^2 * Precision + Recall)
- F0.5-Measure = (1.25 * Precision * Recall) / (0.25 * Precision + Recall)
Consider the case where we have 50 percent precision and perfect recall. We can manually calculate the F0.5-measure for this case as follows:
- F0.5-Measure = (1.25 * Precision * Recall) / (0.25 * Precision + Recall)
- F0.5-Measure = (1.25 * 0.5 * 1.0) / (0.25 * 0.5 + 1.0)
- F0.5-Measure = 0.625 / 1.125
- F0.5-Measure = 0.555
We would expect that a beta value of 0.5 would result in a lower score for this scenario given that precision has a poor score and the recall is excellent.
This is exactly what we see, where an F0.5-measure of 0.555 is achieved for the same scenario where an F1-score was calculated as 0.667. Precision played more of a role in the calculation.
We can confirm this calculation; the complete example is listed below.
# calculate the f0.5-measure from sklearn.metrics import fbeta_score from sklearn.metrics import f1_score from sklearn.metrics import precision_score from sklearn.metrics import recall_score # perfect precision, 50% recall y_true = [0, 0, 0, 0, 0, 1, 1, 1, 1, 1] y_pred = [1, 1, 1, 1, 1, 1, 1, 1, 1, 1] p = precision_score(y_true, y_pred) r = recall_score(y_true, y_pred) f = fbeta_score(y_true, y_pred, beta=0.5) print('Result: p=%.3f, r=%.3f, f=%.3f' % (p, r, f))
Running the example confirms the precision and recall values, then reports an F0.5-measure of 0.556 (with rounding), the same value as we calculated manually.
Result: p=0.500, r=1.000, f=0.556
F2-Measure
The F2-measure is an example of the Fbeta-measure with a beta value of 2.0.
It has the effect of lowering the importance of precision and increase the importance of recall.
If maximizing precision minimizes false positives, and maximizing recall minimizes false negatives, then the F2-measure puts more attention on minimizing false negatives than minimizing false positives.
The F2-measure is calculated as follows:
- F2-Measure = ((1 + 2^2) * Precision * Recall) / (2^2 * Precision + Recall)
- F2-Measure = (5 * Precision * Recall) / (4 * Precision + Recall)
Consider the case where we have 50 percent precision and perfect recall.
We can manually calculate the F2-measure for this case as follows:
- F2-Measure = (5 * Precision * Recall) / (4 * Precision + Recall)
- F2-Measure = (5 * 0.5 * 1.0) / (4 * 0.5 + 1.0)
- F2-Measure = 2.5 / 3.0
- F2-Measure = 0.833
We would expect that a beta value of 2.0 would result in a higher score for this scenario given that recall has a perfect score, which will be promoted over that of the poor performance of precision.
This is exactly what we see where an F2-measure of 0.833 is achieved for the same scenario where an F1-score was calculated as 0.667. Recall played more of a role in the calculation.
We can confirm this calculation; the complete example is listed below.
# calculate the f2-measure from sklearn.metrics import fbeta_score from sklearn.metrics import f1_score from sklearn.metrics import precision_score from sklearn.metrics import recall_score # perfect precision, 50% recall y_true = [0, 0, 0, 0, 0, 1, 1, 1, 1, 1] y_pred = [1, 1, 1, 1, 1, 1, 1, 1, 1, 1] p = precision_score(y_true, y_pred) r = recall_score(y_true, y_pred) f = fbeta_score(y_true, y_pred, beta=2.0) print('Result: p=%.3f, r=%.3f, f=%.3f' % (p, r, f))
Running the example confirms the precision and recall values, then reports an F2-measure of 0.883, the same value as we calculated manually (with rounding).
Result: p=0.500, r=1.000, f=0.833
Further Reading
This section provides more resources on the topic if you are looking to go deeper.
Tutorials
- Tour of Evaluation Metrics for Imbalanced Classification
- How to Calculate Precision, Recall, and F-Measure for Imbalanced Classification
Papers
- The truth of the F-measure, 2007.
APIs
Articles
Summary
In this tutorial, you discovered the Fbeta-measure for evaluating classification algorithms for machine learning.
Specifically, you learned:
- Precision and recall provide two ways to summarize the errors made for the positive class in a binary classification problem.
- F-measure provides a single score that summarizes the precision and recall.
- Fbeta-measure provides a configurable version of the F-measure to give more or less attention to the precision and recall measure when calculating a single score.
Do you have any questions?
Ask your questions in the comments below and I will do my best to answer.
The post A Gentle Introduction to the Fbeta-Measure for Machine Learning appeared first on Machine Learning Mastery.
Ai
via https://www.AiUpNow.com
February 23, 2020 at 01:08PM by Jason Brownlee, Khareem Sudlow