The Leave-One-Out Cross-Validation, or LOOCV, procedure is used to estimate the performance of machine learning algorithms when they are used to make predictions on data not used to train the model.
It is a computationally expensive procedure to perform, although it results in a reliable and unbiased estimate of model performance. Although simple to use and no configuration to specify, there are times when the procedure should not be used, such as when you have a very large dataset or a computationally expensive model to evaluate.
In this tutorial, you will discover how to evaluate machine learning models using leave-one-out cross-validation.
After completing this tutorial, you will know:
- The leave-one-out cross-validation procedure is appropriate when you have a small dataset or when an accurate estimate of model performance is more important than the computational cost of the method.
- How to use the scikit-learn machine learning library to perform the leave-one-out cross-validation procedure.
- How to evaluate machine learning algorithms for classification and regression using leave-one-out cross-validation.
Let’s get started.
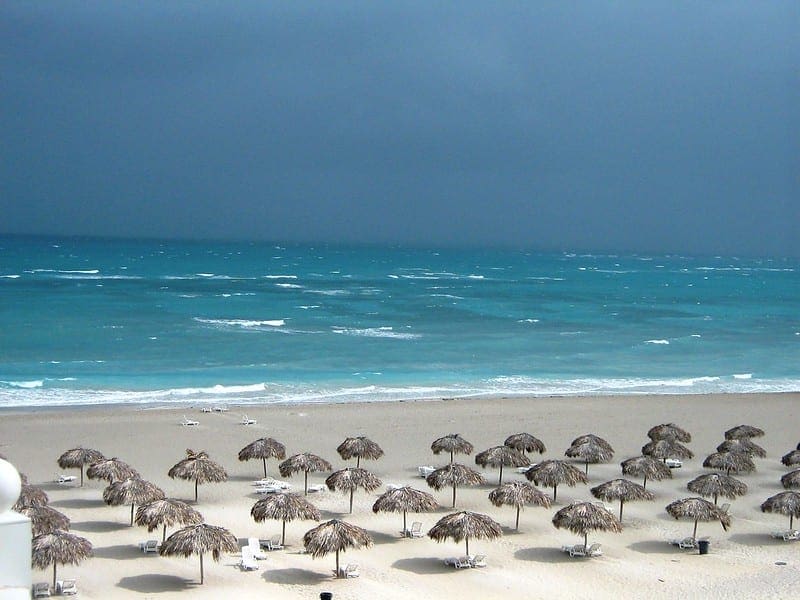
LOOCV for Evaluating Machine Learning Algorithms
Photo by Heather Harvey, some rights reserved.
Tutorial Overview
This tutorial is divided into three parts; they are:
- LOOCV Model Evaluation
- LOOCV Procedure in Scikit-Learn
- LOOCV to Evaluate Machine Learning Models
- LOOCV for Classification
- LOOCV for Regression
LOOCV Model Evaluation
Cross-validation, or k-fold cross-validation, is a procedure used to estimate the performance of a machine learning algorithm when making predictions on data not used during the training of the model.
The cross-validation has a single hyperparameter “k” that controls the number of subsets that a dataset is split into. Once split, each subset is given the opportunity to be used as a test set while all other subsets together are used as a training dataset.
This means that k-fold cross-validation involves fitting and evaluating k models. This, in turn, provides k estimates of a model’s performance on the dataset, which can be reported using summary statistics such as the mean and standard deviation. This score can then be used to compare and ultimately select a model and configuration to use as the “final model” for a dataset.
Typical values for k are k=3, k=5, and k=10, with 10 representing the most common value. This is because, given extensive testing, 10-fold cross-validation provides a good balance of low computational cost and low bias in the estimate of model performance as compared to other k values and a single train-test split.
For more on k-fold cross-validation, see the tutorial:
Leave-one-out cross-validation, or LOOCV, is a configuration of k-fold cross-validation where k is set to the number of examples in the dataset.
LOOCV is an extreme version of k-fold cross-validation that has the maximum computational cost. It requires one model to be created and evaluated for each example in the training dataset.
The benefit of so many fit and evaluated models is a more robust estimate of model performance as each row of data is given an opportunity to represent the entirety of the test dataset.
Given the computational cost, LOOCV is not appropriate for very large datasets such as more than tens or hundreds of thousands of examples, or for models that are costly to fit, such as neural networks.
- Don’t Use LOOCV: Large datasets or costly models to fit.
Given the improved estimate of model performance, LOOCV is appropriate when an accurate estimate of model performance is critical. This particularly case when the dataset is small, such as less than thousands of examples, can lead to model overfitting during training and biased estimates of model performance.
Further, given that no sampling of the training dataset is used, this estimation procedure is deterministic, unlike train-test splits and other k-fold cross-validation confirmations that provide a stochastic estimate of model performance.
- Use LOOCV: Small datasets or when estimated model performance is critical.
Once models have been evaluated using LOOCV and a final model and configuration chosen, a final model is then fit on all available data and used to make predictions on new data.
Now that we are familiar with the LOOCV procedure, let’s look at how we can use the method in Python.
LOOCV Procedure in Scikit-Learn
The scikit-learn Python machine learning library provides an implementation of the LOOCV via the LeaveOneOut class.
The method has no configuration, therefore, no arguments are provided to create an instance of the class.
... # create loocv procedure cv = LeaveOneOut()
Once created, the split() function can be called and provided the dataset to enumerate.
Each iteration will return the row indices that can be used for the train and test sets from the provided dataset.
... for train_ix, test_ix in cv.split(X): ...
These indices can be used on the input (X) and output (y) columns of the dataset array to split the dataset.
... # split data X_train, X_test = X[train_ix, :], X[test_ix, :] y_train, y_test = y[train_ix], y[test_ix]
The training set can be used to fit a model and the test set can be used to evaluate it by first making a prediction and calculating a performance metric on the predicted values versus the expected values.
... # fit model model = RandomForestClassifier(random_state=1) model.fit(X_train, y_train) # evaluate model yhat = model.predict(X_test)
Scores can be saved from each evaluation and a final mean estimate of model performance can be presented.
We can tie this together and demonstrate how to use LOOCV to evaluate a RandomForestClassifier model for a synthetic binary classification dataset created with the make_blobs() function.
The complete example is listed below.
# loocv to manually evaluate the performance of a random forest classifier from sklearn.datasets import make_blobs from sklearn.model_selection import LeaveOneOut from sklearn.ensemble import RandomForestClassifier from sklearn.metrics import accuracy_score # create dataset X, y = make_blobs(n_samples=100, random_state=1) # create loocv procedure cv = LeaveOneOut() # enumerate splits y_true, y_pred = list(), list() for train_ix, test_ix in cv.split(X): # split data X_train, X_test = X[train_ix, :], X[test_ix, :] y_train, y_test = y[train_ix], y[test_ix] # fit model model = RandomForestClassifier(random_state=1) model.fit(X_train, y_train) # evaluate model yhat = model.predict(X_test) # store y_true.append(y_test[0]) y_pred.append(yhat[0]) # calculate accuracy acc = accuracy_score(y_true, y_pred) print('Accuracy: %.3f' % acc)
Running the example manually estimates the performance of the random forest classifier on the synthetic dataset.
Given that the dataset has 100 examples, it means that 100 train/test splits of the dataset were created, with each single row of the dataset given an opportunity to be used as the test set. Similarly, 100 models are created and evaluated.
The classification accuracy across all predictions is then reported, in this case as 99 percent.
Accuracy: 0.990
A downside of enumerating the folds manually is that it is slow and involves a lot of code that could introduce bugs.
An alternative to evaluating a model using LOOCV is to use the cross_val_score() function.
This function takes the model, the dataset, and the instantiated LOOCV object set via the “cv” argument. A sample of accuracy scores is then returned that can be summarized by calculating the mean and standard deviation.
We can also set the “n_jobs” argument to -1 to use all CPU cores, greatly decreasing the computational cost in fitting and evaluating so many models.
The example below demonstrates evaluating the RandomForestClassifier using LOOCV on the same synthetic dataset using the cross_val_score() function.
# loocv to automatically evaluate the performance of a random forest classifier from numpy import mean from numpy import std from sklearn.datasets import make_blobs from sklearn.model_selection import LeaveOneOut from sklearn.model_selection import cross_val_score from sklearn.ensemble import RandomForestClassifier # create dataset X, y = make_blobs(n_samples=100, random_state=1) # create loocv procedure cv = LeaveOneOut() # create model model = RandomForestClassifier(random_state=1) # evaluate model scores = cross_val_score(model, X, y, scoring='accuracy', cv=cv, n_jobs=-1) # report performance print('Accuracy: %.3f (%.3f)' % (mean(scores), std(scores)))
Running the example automatically estimates the performance of the random forest classifier on the synthetic dataset.
The mean classification accuracy across all folds matches our manual estimate previously.
Accuracy: 0.990 (0.099)
Now that we are familiar with how to use the LeaveOneOut class, let’s look at how we can use it to evaluate a machine learning model on real datasets.
LOOCV to Evaluate Machine Learning Models
In this section, we will explore using the LOOCV procedure to evaluate machine learning models on standard classification and regression predictive modeling datasets.
LOOCV for Classification
We will demonstrate how to use LOOCV to evaluate a random forest algorithm on the sonar dataset.
The sonar dataset is a standard machine learning dataset comprising 208 rows of data with 60 numerical input variables and a target variable with two class values, e.g. binary classification.
The dataset involves predicting whether sonar returns indicate a rock or simulated mine.
No need to download the dataset; we will download it automatically as part of our worked examples.
The example below downloads the dataset and summarizes its shape.
# summarize the sonar dataset from pandas import read_csv # load dataset url = 'https://raw.githubusercontent.com/jbrownlee/Datasets/master/sonar.csv' dataframe = read_csv(url, header=None) # split into input and output elements data = dataframe.values X, y = data[:, :-1], data[:, -1] print(X.shape, y.shape)
Running the example downloads the dataset and splits it into input and output elements. As expected, we can see that there are 208 rows of data with 60 input variables.
(208, 60) (208,)
We can now evaluate a model using LOOCV.
First, the loaded dataset must be split into input and output components.
... # split into inputs and outputs X, y = data[:, :-1], data[:, -1] print(X.shape, y.shape)
Next, we define the LOOCV procedure.
... # create loocv procedure cv = LeaveOneOut()
We can then define the model to evaluate.
... # create model model = RandomForestClassifier(random_state=1)
Then use the cross_val_score() function to enumerate the folds, fit models, then make and evaluate predictions. We can then report the mean and standard deviation of model performance.
... # evaluate model scores = cross_val_score(model, X, y, scoring='accuracy', cv=cv, n_jobs=-1) # report performance print('Accuracy: %.3f (%.3f)' % (mean(scores), std(scores)))
Tying this together, the complete example is listed below.
# loocv evaluate random forest on the sonar dataset from numpy import mean from numpy import std from pandas import read_csv from sklearn.model_selection import LeaveOneOut from sklearn.model_selection import cross_val_score from sklearn.ensemble import RandomForestClassifier # load dataset url = 'https://raw.githubusercontent.com/jbrownlee/Datasets/master/sonar.csv' dataframe = read_csv(url, header=None) data = dataframe.values # split into inputs and outputs X, y = data[:, :-1], data[:, -1] print(X.shape, y.shape) # create loocv procedure cv = LeaveOneOut() # create model model = RandomForestClassifier(random_state=1) # evaluate model scores = cross_val_score(model, X, y, scoring='accuracy', cv=cv, n_jobs=-1) # report performance print('Accuracy: %.3f (%.3f)' % (mean(scores), std(scores)))
Running the example first loads the dataset and confirms the number of rows in the input and output elements.
The model is then evaluated using LOOCV and the estimated performance when making predictions on new data has an accuracy of about 82.2 percent.
(208, 60) (208,) Accuracy: 0.822 (0.382)
LOOCV for Regression
We will demonstrate how to use LOOCV to evaluate a random forest algorithm on the housing dataset.
The housing dataset is a standard machine learning dataset comprising 506 rows of data with 13 numerical input variables and a numerical target variable.
The dataset involves predicting the house price given details of the house’s suburb in the American city of Boston.
No need to download the dataset; we will download it automatically as part of our worked examples.
The example below downloads and loads the dataset as a Pandas DataFrame and summarizes the shape of the dataset.
# load and summarize the housing dataset from pandas import read_csv # load dataset url = 'https://raw.githubusercontent.com/jbrownlee/Datasets/master/housing.csv' dataframe = read_csv(url, header=None) # summarize shape print(dataframe.shape)
Running the example confirms the 506 rows of data and 13 input variables and single numeric target variables (14 in total).
(506, 14)
We can now evaluate a model using LOOCV.
First, the loaded dataset must be split into input and output components.
... # split into inputs and outputs X, y = data[:, :-1], data[:, -1] print(X.shape, y.shape)
Next, we define the LOOCV procedure.
... # create loocv procedure cv = LeaveOneOut()
We can then define the model to evaluate.
... # create model model = RandomForestRegressor(random_state=1)
Then use the cross_val_score() function to enumerate the folds, fit models, then make and evaluate predictions. We can then report the mean and standard deviation of model performance.
In this case, we use the mean absolute error (MAE) performance metric appropriate for regression.
... # evaluate model scores = cross_val_score(model, X, y, scoring='neg_mean_absolute_error', cv=cv, n_jobs=-1) # force positive scores = absolute(scores) # report performance print('MAE: %.3f (%.3f)' % (mean(scores), std(scores)))
Tying this together, the complete example is listed below.
# loocv evaluate random forest on the housing dataset from numpy import mean from numpy import std from numpy import absolute from pandas import read_csv from sklearn.model_selection import LeaveOneOut from sklearn.model_selection import cross_val_score from sklearn.ensemble import RandomForestRegressor # load dataset url = 'https://raw.githubusercontent.com/jbrownlee/Datasets/master/housing.csv' dataframe = read_csv(url, header=None) data = dataframe.values # split into inputs and outputs X, y = data[:, :-1], data[:, -1] print(X.shape, y.shape) # create loocv procedure cv = LeaveOneOut() # create model model = RandomForestRegressor(random_state=1) # evaluate model scores = cross_val_score(model, X, y, scoring='neg_mean_absolute_error', cv=cv, n_jobs=-1) # force positive scores = absolute(scores) # report performance print('MAE: %.3f (%.3f)' % (mean(scores), std(scores)))
Running the example first loads the dataset and confirms the number of rows in the input and output elements.
The model is evaluated using LOOCV and the performance of the model when making predictions on new data is a mean absolute error of about 2.180 (thousands of dollars).
(506, 13) (506,) MAE: 2.180 (2.346)
Further Reading
This section provides more resources on the topic if you are looking to go deeper.
Tutorials
APIs
- Cross-validation: evaluating estimator performance, scikit-learn.
- sklearn.model_selection.LeaveOneOut API.
- sklearn.model_selection.cross_val_score API.
Summary
In this tutorial, you discovered how to evaluate machine learning models using leave-one-out cross-validation.
Specifically, you learned:
- The leave-one-out cross-validation procedure is appropriate when you have a small dataset or when an accurate estimate of model performance is more important than the computational cost of the method.
- How to use the scikit-learn machine learning library to perform the leave-one-out cross-validation procedure.
- How to evaluate machine learning algorithms for classification and regression using leave-one-out cross-validation.
Do you have any questions?
Ask your questions in the comments below and I will do my best to answer.
The post LOOCV for Evaluating Machine Learning Algorithms appeared first on Machine Learning Mastery.
Ai
via https://www.AiUpNow.com
July 26, 2020 at 03:12PM by Jason Brownlee, Khareem Sudlow